From data to knowledge: the evolution of analytics
Analytics is about augmenting and automating the process of going from data to knowledge to inform action1. Data is the raw records underpinning operational applications, while information is the raw data processed and restructured to enable more meaningful inquiry2. The insights from this inquiry are the knowledge that moves organizations forward through more informed decision-making and the resulting intentional action3.
In 2013, Gartner published the diagram below, articulating the continuum of data, information and knowledge and the evolution of analytics in this continuum4. The diagram shows how enabling higher-quality decisions and actions deduced from data at scale reduces the need for human intervention and also makes the interfaces between computers and the environment more opaque.
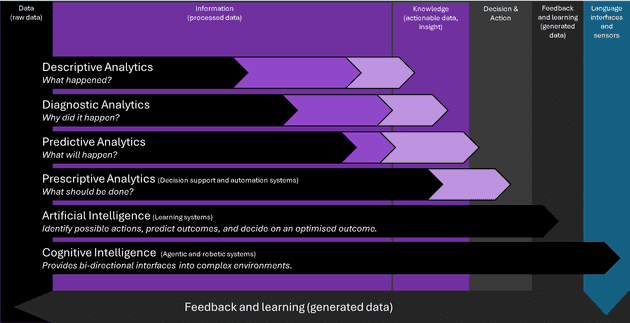
Discriptive and Diagnostic analytics
The first-wave analytics projects promised that organizations could transform data into information by deploying solutions optimized for data analysis alongside operational applications. A proper extract, transform, and load (ETL) process and the appropriate reporting solution promise access to the information signals buried in operational data. Organizations can then apply their best strategic, analytical, and critical thinking to these information signals to surface knowledge insights that improve decision-making and action. This process is called descriptive analytics5.
In an insurance company, we might answer several questions using descriptive analytics, such as: "How much premium have we earned over the past month for a specific product?" "Which state generated the highest premium?" "How many policies have had claims filed against them?" or "What is our total liability for a specific coverage in a particular state?".
As we look deeper into historical data, we will naturally start to ask diagnostic questions such as: "What factors determine which policies are claimed?". In response to this question, Excel magicians might conjure pivot tables that subset the historical data across various dimensions. They might find the claim ratio varies meaningfully across state. Similarly, Data scientists might run a regression analysis across multiple dimensions. This analysis might find various factors with significant correlations to the claim ratio. For example, they might discover that the insured's age and state are highly correlated with claims in auto policies. This process of interrogating data to surface information and knowledge might be called diagnostic analytics.
Prescriptive and Predictive Analytics
The second wave of analytics projects promises to bridge the gap between information to knowledge by adding data mining tools (now called machine learning) to the solutions.
In the insurance example, once we identify the factors that correlate with the claim ratio, we can use these factors to predict the probability of a claim being made against a policy. This knowledge can then be used to appriopriately price an issurance policy. This process can be referred to as predictive analytics. By taking measurable attributes before the fact, such as an insured's age and location, we can forecast outcomes which can only be assessed after they occur, such as the likelihood of a claim being made against a policy. In insurance companies, we develop sophisticated model ensables that not only predict the occurrence of a claim but also estimate the potential value of that claim. This predictive modeling forms the core of the underwriting process, allowing for a more informed risk assessment, risk pricing and risk portfolio management.
The extraction of knowledge from information and data helps create value accross most knowledge organizations. Prescriptive analytics may leverage tools from the statitical tool box such as logistic regression or computational toolbox such as multi-layer perceptron neural networks. Once a reliable prescritive model is in place, an organization may begin to integrate it into their business processes. This integration supports and automates decision-making at scale.
In an insurnace company, we can embed underwriting decisions directly into our retail B2C sales channels. In this scenario, we ask our retail customers tailored questions about themselves, and use their responses to predict the quality of the risk they present. This approach is known as prescriptive analytics, helping to streamline our operations and enhance our decision-making capabilities.
Artficial and Congitive intelligence
You might have come across the notion of MLOps, which involves how organizations deploy, monitor and maintain prescriptive analytics as part of software solutions. Historically, when organizations embed prescriptive analytics into digital solutions to support and automate decision-making they were called artificial intelligent (AI) solutions. AI is an advanced analytics capability that not only aims to surfaces knowledge from data and information, but also to use this knowledge to make decisions and take action against in a way that optimizes desired outcomes. Most importantly AI is learning systems.
The latest wave of advanced analytics solutions promises to further bridge the gap between knowledge, decision, action and the environement. These systems sense the actual outcome of decision while maintaining feedback loops that improve future decision-action cycles. The interface between these systems and the environments in which they operate is also blured.
Notes
- This post is part of a series. See here.
Footnotes and references
Footnotes
-
The Institute for Operations Research and the Management Sciences (INFORMS) defines analytics as "the scientific process of transforming data into insight for making better decisions. ↩
-
Data is raw facts, numbers, or symbols without inherent meaning or context. Information is the result of processing and organizing data to convey meaning, ↩
-
Knowledge is insights that enable informed decisions, actions, or predictions. Knowledge emerges from applying experience to data. ↩
-
See here. Gartner didn't include artificial and cognitive intelligence. Differentiating between systems that can learn and systems that can also perceive and communicate in our natural world is useful. ↩
-
Check out "What Is Data and Analytics: Everything You Need to Know" by Gartner. ↩
Related tags